Howard University’s Artificial Intelligence and Machine Learning Symposium spotlighted the wide-ranging impacts of this new technology in all fields of science. In particular, two presentations by Howard researchers at the symposium showcased how machine learning is helping researchers better understand the brain, down to the smallest cellular detail. This research could revolutionize our understanding of how the brain functions and lead to faster, more accurate diagnoses and treatment of disease.
Super-Resolve Imaging Using AI May Help Detect Disease Early
Dr. Kofi Deh, a researcher in the Howard’s Department of Physics and Astronomy is collaborating with the Molecular Imaging Laboratory to focus on imaging changes in metabolism — the chemical processes that allow cells to function. These changes can be one of the first indicators of cancer, diabetes, neurological disorders, and a range of other diseases, often occurring long before structural cell changes begin. However, current imaging methods like PET scans and MRI present several challenges, including an inability to see the entire metabolic process, low specificity, and cost. As the models used for image quality improvement and analysis further improve, AI will become more critical to quickly and accurately identifying abnormalities and metabolic changes, leading to faster, more precise diagnoses and treatment.
Deh’s research improves image visual quality by using an artificial neural network to “super-resolve” scans. This requires both natural high-quality images and data on the physical processes being imaged.
“To acquire the higher resolution images that you need for training the neural network, you have to resort to physics-informed methods, where you actually model the processes you are trying to super-resolve,” Deh said.
Deh gave the example of measuring diffusion — the gradual spreading out of molecules from areas of high concentration to low concentration — within human brains.
“You cannot measure diffusion at each point in the brain,” he explained. “What you can do, though, is measure pressure at several points in the brain, or at several arteries. And when you do that you can set up a number of equations which model blood flow.”
These equations can then be used, along with anatomical images, by the neural network to help ensure images are enhanced accurately.
Mapping Brain Behavior Through Machine Learning
Dr. Dominique Pritchett, an associate professor in the Department of Biology, has been working with researchers from Howard, the University of Michigan, Stanford University, and the Army Research Lab to capture the complex dynamics of whole-brain behavior, in which each part of the brain operates codependently with other parts. One area of his research has been to examine the effect of anesthesia on the brain, and then use that information to explore broader implications related to how other substances, environments, or body states may impact the brain’s integrated functions.
His current research focuses on zebrafish, a species that not only is highly genetically malleable and fast-breeding but also transparent, making them ideal subjects for genetics and neuroscience research.
“That transparency allows us to illuminate the brain from the surface and actually [see?] what’s happening across many, many neurons simultaneously,” said Pritchett.
His current research focuses on the effects of propofol anesthesia — a drug that enhances the activity of GABA, a key inhibitory neurotransmitter — on neural dynamics in zebrafish. Using light-sheet microscopy, Pritchett and his colleagues can illuminate and image thousands of cells in real-time, capturing the activity of neurons during different behavioral states.
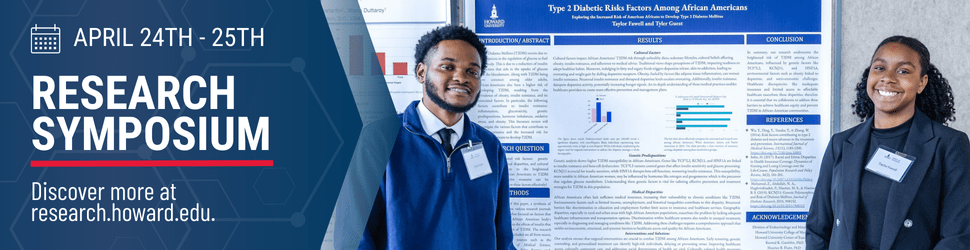
This imaging has led to a massive amount of data on how different brain regions react to anesthesia during different behaviors — data that is then used by the Computer Science Lab to develop machine learning foundational models based on how the brain operates. Given the size of the samples and recordings, machine learning has also been critical to analysis and allowed the researchers to segment out changes across individual cells.
In addition to deepening our understanding of how brain regions interact during altered states, Pritchett’s research may shed new light on how medications affect neural circuits, offering unprecedented detail at the cellular level.